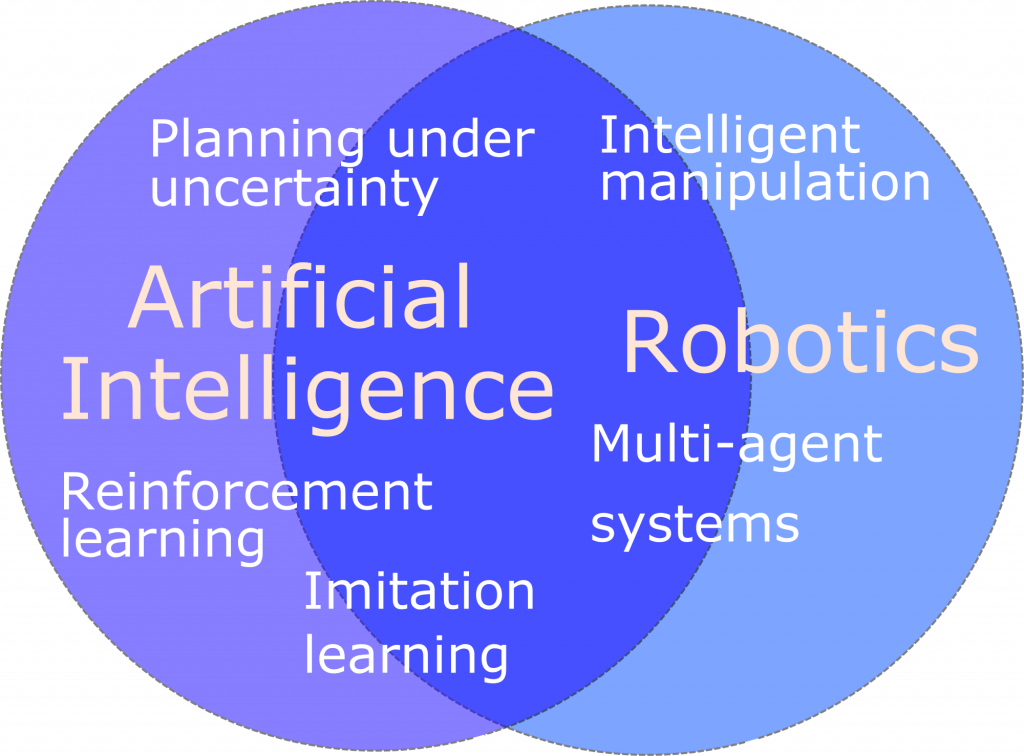
The Learning for Intelligent Autonomous Robots (LIAR) research group
operates in the intersection of artificial intelligence and robotics. We focus on reinforcement learning, robotic manipulation, decision making under partial observability, imitation learning, and decision making in multi-agent systems
Our future vision is a world where autonomous robots can enter an unknown unstructured environment, clean up toys, arrange objects in the kitchen, segregate waste, or pick up and lift tree trunks onto a truck. To accomplish this, in a partially unknown environment a rational robot needs to plan its actions so that the robot learns about the environment while performing its assigned tasks. This can be especially challenging in environments where many objects occlude each other, such as when segregating waste or in heterogeneous environments, such as homes or in agile manufacturing.
The goal of the research group is to help robots understand what they need to learn in order to perform their assigned tasks, and, thus, make robots capable of operating on their own and pro-actively help humans. To accomplish these goals the research group develops novel decision making methods and uses these methods to solve unsolved robotic tasks.
In particular, we focus on the development of computational methods and algorithms for reinforcement learning, planning under uncertainty, and decision making in multi-agent systems.
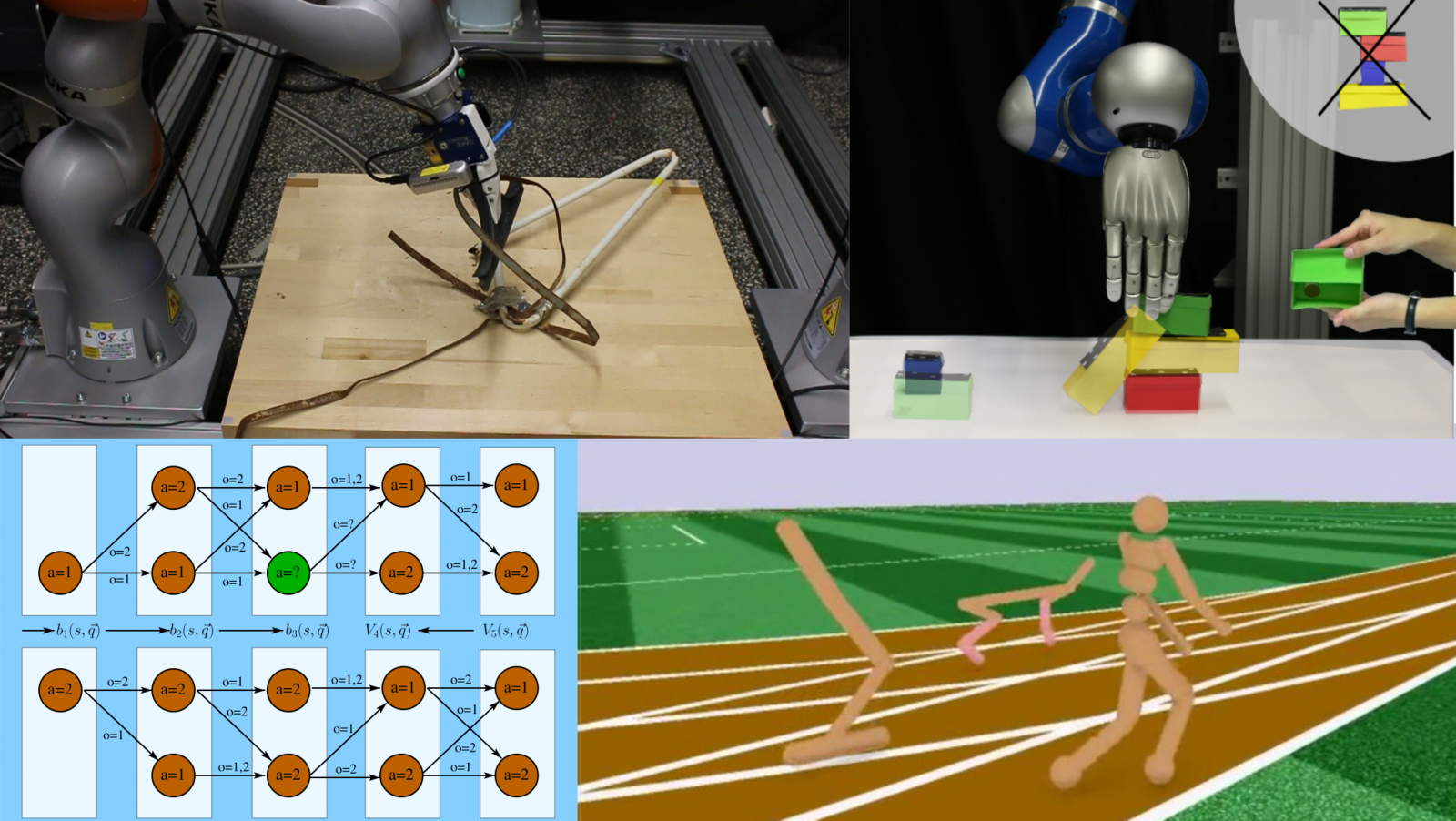