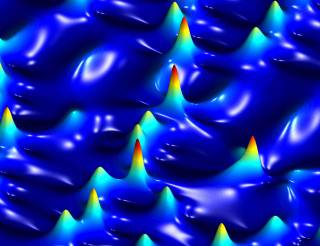
Machine learning is an umbrella term that describes the use of statistical techniques for data analysis applied to detect patterns and predict future outcomes. Machine learning algorithms is commonly divided into two classes of algorithms depending on the approach used for analysis: supervised and unsupervised learning. In supervised learning, knowledge of the relation between the input and output of a system is used to build a model that relates the input and output. In unsupervised learning, the analysis is more exploratory and an algorithm is typically searching for patterns and structures in a data ensemble without any particular knowledge about the system.
We use both unsupervised and supervised learning strategies to analyse and model nonlinear instabilities and propagation dynamics in optical fiber systems. Our studies include both noise-seeded processes as well as coherently seeded dynamics and have applications in the study and control ultrafast transient dynamics as well as in the design of smart laser sources with on-demand properties.
[1] Genty, G., Salmela, L., Dudley, J.M. et al. Machine learning and applications in ultrafast photonics. Nat. Photonics 15, 91–101 (2021). link
[2] Närhi, M., Salmela, L., Toivonen, J. et al. Machine learning analysis of extreme events in optical fibre modulation instability. Nat Commun 9, 4923 (2018). link
[3] Salmela, L., Tsipinakis, N., Foi, A. et al. Predicting ultrafast nonlinear dynamics in fibre optics with a recurrent neural network. Nat Mach Intell 3, 344–354 (2021). link
[4] Salmela, L., Lapre, C., Dudley, J.M. et al. Machine learning analysis of rogue solitons in supercontinuum generation. Sci Rep 10, 9596 (2020). link
[5] Mathilde Hary, Lauri Salmela, Piotr Ryczkowski, Francesca Gallazzi, John M. Dudley, and Goëry Genty, “Tailored supercontinuum generation using genetic algorithm optimized Fourier domain pulse shaping,” Opt. Lett. 48, 4512-4515 (2023) . link