We perform experimental, numerical and theoretical studies of ultrafast phenomena in optics. Our research includes the characterization and control in real-time of ultrafast laser dynamics and nonlinear light-matter interactions, the development of ultra-broadband light sources with tailored characteristics, the development of novel correlation sensing and imaging approaches as well as the application of machine learning to the design of smart laser sources. Our expertise ranges from the design and optimisation of broadband supercontinuum light sources, to advanced real-time characterization methods, computational imaging, and ultrafast photonics application of machine learning.
Studies of Ultrafast Nonlinear Dynamics
Instabilities and nonlinear dynamics are central to nonlinear science. We develop and apply advanced methods for the real-time measurement of ultrafast dynamics and instabilities in lasers and optical fibers.
Supercontinuum Generation
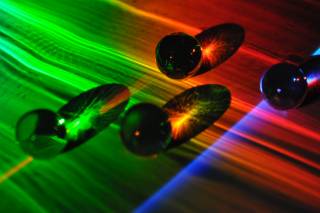
The propagation of an intense and short pulse in an optical fiber or waveguide can lead to massive spectral broadening of the initial pulse as a result of cascaded nonlinear light-matter interactions. These effects can be efficiently harnessed and applied to the generation of light source with an ultra-broadband supercontinuum spectrum. Supercontinuum light sources often referred to as white-light lasers have unique properties in terms of brightness, coherence and wavelength coverage. They have had major impact in many research fields in the past two decades ranging from precision metrology, ultrafast spectroscopy, and the calibration of astro-combs, to high resolution imaging, LIDAR technologies, and the generation of ultrastable microwave signals. Our group has a long history of research into the modelling, development and applications of supercontinuum light sources. Recently, our research have focused onto high-power mid-infrared supercontinuum studies in multimode soft-glass fibers and gradient-index fibers. We are also developing supercontiuum sources for applications in optical imaging, sensing and spectroscopy.
Machine Learning Applications to Ultrafast Photonics
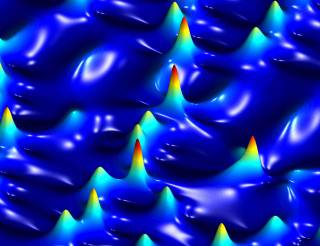
Machine learning is an umbrella term that describes the use of statistical techniques for data analysis applied to detect patterns and predict future outcomes. Machine learning algorithms is commonly divided into two classes of algorithms depending on the approach used for analysis: supervised and unsupervised learning. In supervised learning, knowledge of the relation between the input and output of a system is used to build a model that relates the input and output. In unsupervised learning, the analysis is more exploratory and an algorithm is typically searching for patterns and structures in a data ensemble without any particular knowledge about the system.
We use both unsupervised and supervised learning strategies to analyse and model nonlinear instabilities and propagation dynamics in optical fiber systems. Our studies include both noise-seeded processes as well as coherently seeded dynamics and have applications in the study and control ultrafast transient dynamics as well as in the design of smart laser sources with on-demand properties.
Correlation Imaging
We develop computational and correlation imaging methods in the temporal and spectral domain.