The article "Efficient Image-Warping Framework for Content-Adaptive Superpixels Generation" by Aleksandra Chuchvara and Atanas Gotchev has been accepted for publication in a future issue of IEEE Signal Processing Letters and is available under the "Early Access" area on IEEE Xplore.
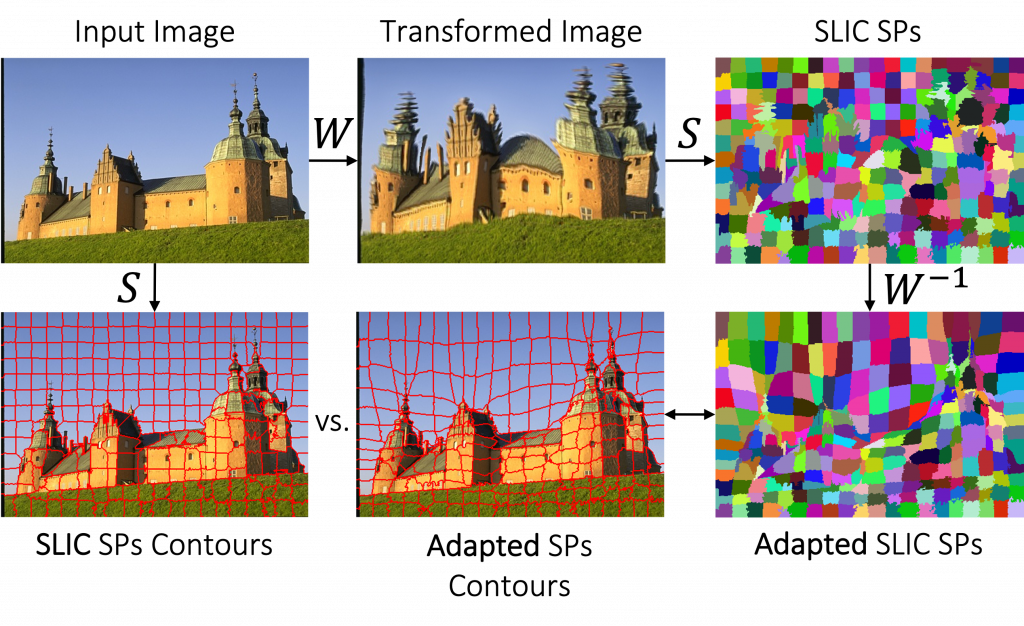
Abstract:
We address the problem of efficient content-adaptive superpixel segmentation. Instead of adapting the size and/or amount of superpixels to the image content, we propose a warping transform that makes the image content more suitable to be segmented into regular superpixels. Regular superpixels in the warped image induce content-adaptive superpixels in the original image with improved segmentation accuracy. To efficiently compute the warping transform, we develop an iterative coarse-to-fine optimization procedure and employ a parallelization strategy allowing for a speedy GPU-based implementation. The proposed solution works as a simple add-on framework over an underlying segmentation algorithm and requires no additional parameters. Evaluations on the Berkeley segmentation dataset verify that our approach provides competitive quality results compared to the state-of-the-art methods and achieves a better time-accuracy trade-off. We further demonstrate the effectiveness of our method with an application to disparity estimation.